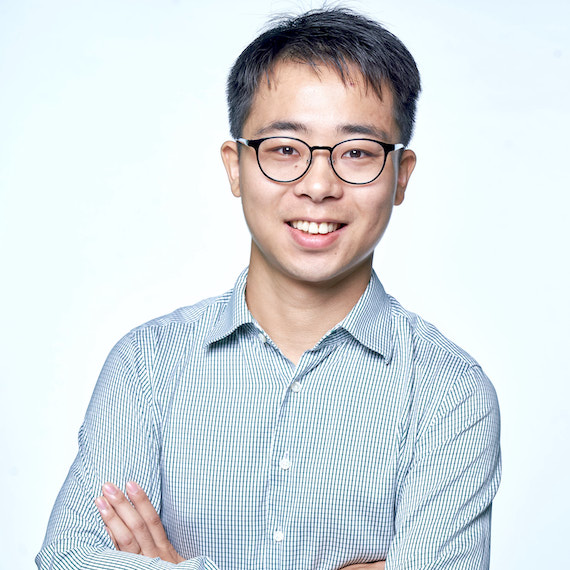
Frank Xing, PhD | 邢竹天
Assistant Professor
Department of Information Systems and Analytics
School of Computing
National University of Singapore
Where to find: (physically) COM2-03-39, or (online) xing(ατ)nus.edu.sg. [
calendar] [
curriculum vitae]
News
※ Dec 2023: See you in Hyderabad ;)
※ May 2023: Three undergrad students successfully defended their theses on financial text mining and algorithmic trading. Congratulations to them!
About me
I am, broadly speaking, an information scientist. I lived in Wuhan, Beijing, and Hong Kong before moving to Singapore.
I spend time teaching, thinking, and being with family.
Fun facts
※ My academic genealogy (observation from history: don't be afraid to cross over) [click here to expand].
Jim Nightingale (1959, prosthetic engineer)
Stanley Parker Smith (1907, electrical engineer)
Andreas von Ettingshausen (1817, mathematician)
Edwin Williams (1974, linguist)
James Alan Montgomery (1904, orientalist)
Hermann Volrath Hilprecht (1883, archaeologist)
Ferdinand von Lindemann (1873, mathematician)
※ My Erdös number is 3 and Davis number is 4.